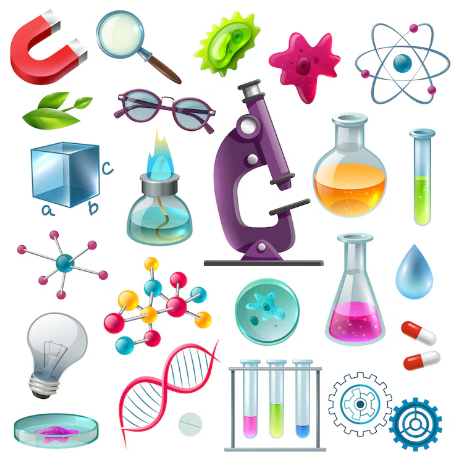
Introduction:
In the modern era, the proliferation of digital technologies has led to an explosion in data generation across various industries and domains. This unprecedented volume of data, often referred to as Big Data, holds immense potential for organizations to gain valuable insights, optimize processes, and drive innovation. In this comprehensive exploration of Big Data technology, we delve into its underlying principles, applications, challenges, and future prospects, shedding light on how it is reshaping the landscape of business, science, and society.
Understanding Big Data:
- Volume: At the core of Big Data lies its sheer volume, encompassing datasets of immense size that traditional data processing tools struggle to handle efficiently. These datasets can range from terabytes to petabytes and beyond, comprising structured, semi-structured, and unstructured data from diverse sources such as social media, sensor networks, transaction records, and scientific research. Managing, storing, and processing such massive volumes of data require scalable infrastructure and distributed computing technologies capable of parallel processing and storage.
- Velocity: In addition to volume, Big Data is characterized by its velocity, referring to the speed at which data is generated, collected, and processed. With the advent of IoT devices, social media platforms, and online transactions, data streams continuously flow into systems at unprecedented rates, demanding real-time or near-real-time processing capabilities. Stream processing technologies enable organizations to analyze data as it is generated, facilitating timely decision-making and response to dynamic events and trends.
- Variety: Big Data exhibits a variety of data types and formats, including structured data stored in relational databases, semi-structured data such as XML and JSON, and unstructured data such as text documents, images, and videos. Traditional relational databases are ill-suited to handle the diversity and complexity of Big Data, necessitating the use of NoSQL databases, Hadoop Distributed File System (HDFS), and object storage systems that can accommodate heterogeneous data formats and scale horizontally.
- Veracity: Veracity refers to the reliability, accuracy, and trustworthiness of data, which can vary widely in Big Data environments. Data sources may contain errors, inconsistencies, or biases that impact the quality and validity of analytical results. Data quality management practices, including data cleansing, deduplication, and validation, are essential for ensuring the integrity of data throughout its lifecycle. Advanced analytics techniques such as anomaly detection and outlier analysis help identify and mitigate data quality issues, enabling organizations to make informed decisions based on reliable data.
Applications of Big Data Technology:
- Business Intelligence and Analytics: Big Data technology enables organizations to extract actionable insights from large volumes of data, driving business intelligence and analytics initiatives. Data warehousing, data mining, and predictive analytics techniques uncover patterns, trends, and correlations in data, empowering businesses to optimize operations, identify market opportunities, and mitigate risks. Business intelligence dashboards and visualization tools transform complex data into intuitive visualizations, facilitating data-driven decision-making and strategic planning.
- Customer Relationship Management (CRM): Big Data technology revolutionizes customer relationship management (CRM) by capturing, analyzing, and leveraging customer data to personalize interactions and enhance customer experiences. Customer data platforms (CDPs) aggregate data from multiple sources, including transaction records, website visits, and social media interactions, to create unified customer profiles. Machine learning algorithms enable organizations to segment customers, identify buying patterns, and tailor marketing campaigns and product recommendations to individual preferences, driving customer engagement and loyalty.
- Healthcare and Life Sciences: In healthcare and life sciences, Big Data technology transforms patient care, medical research, and drug discovery processes. Electronic health records (EHRs), medical imaging data, and genomic data provide valuable insights into patient health, disease patterns, and treatment outcomes. Big Data analytics platforms analyze large-scale healthcare datasets to identify trends, predict disease outbreaks, and optimize clinical workflows. Machine learning algorithms assist in diagnosing diseases, identifying personalized treatment options, and discovering novel drug targets, leading to improved patient outcomes and medical breakthroughs.
- Smart Cities and Urban Planning: Big Data technology plays a pivotal role in building smart cities and optimizing urban infrastructure and services. IoT sensors, mobile devices, and smart meters generate vast amounts of data about traffic patterns, air quality, energy consumption, and public transportation usage. Big Data analytics platforms process and analyze urban data to optimize city operations, reduce traffic congestion, improve public safety, and enhance environmental sustainability. Predictive modeling and simulation techniques enable urban planners to anticipate future trends and make data-driven decisions to shape the future of cities.
Challenges and Considerations in Big Data Technology:
- Data Privacy and Security: As organizations collect and analyze large volumes of data, protecting data privacy and ensuring security become paramount concerns. Data breaches, unauthorized access, and data misuse pose significant risks to sensitive information and erode consumer trust. Compliance with data protection regulations, such as GDPR and CCPA, requires organizations to implement robust data privacy and security measures, including encryption, access controls, and data anonymization techniques, to safeguard data against unauthorized access or disclosure.
- Scalability and Performance: Scalability and performance are critical considerations in Big Data technology, as organizations strive to process and analyze ever-growing volumes of data in a timely and efficient manner. Traditional data processing tools and relational databases may struggle to scale horizontally and handle the complexities of Big Data workloads. Distributed computing frameworks, such as Apache Hadoop, Apache Spark, and Kubernetes, enable organizations to scale out horizontally across clusters of commodity hardware and leverage parallel processing to accelerate data processing tasks.
- Data Governance and Compliance: Effective data governance frameworks are essential for managing data quality, ensuring regulatory compliance, and mitigating risks in Big Data environments. Data governance policies define data ownership, access controls, and data lifecycle management practices to ensure data integrity and accountability. Compliance with data protection regulations, industry standards, and internal policies requires organizations to implement data governance controls, audit trails, and data lineage tracking mechanisms to maintain trust and transparency in data management practices.
Future Prospects in Big Data Technology:
- Artificial Intelligence and Machine Learning Integration: The integration of Artificial Intelligence (AI) and Machine Learning (ML) technologies with Big Data platforms enhances data processing, analytics, and decision-making capabilities. AI-driven data analytics tools automate data discovery, pattern recognition, and anomaly detection tasks, enabling organizations to derive actionable insights from large volumes of data in real-time. ML algorithms enable predictive modeling, recommendation systems, and prescriptive analytics, empowering organizations to anticipate future trends, optimize operations, and drive innovation.
- Edge Computing and IoT Convergence: The convergence of Edge Computing and Internet of Things (IoT) technologies with Big Data platforms extends data processing and analytics capabilities to the network edge. Edge Computing devices, such as IoT sensors, gateways, and edge servers, preprocess and analyze data locally before transmitting aggregated insights to centralized data centers or cloud environments. Edge analytics enable real-time decision-making, reduced latency, and bandwidth optimization for IoT applications, such as smart cities, autonomous vehicles, and industrial automation.
- Quantum Computing for Big Data Analytics: The emergence of Quantum Computing holds promise for revolutionizing Big Data analytics and unlocking new frontiers in data processing and optimization. Quantum algorithms, such as quantum machine learning, quantum optimization, and quantum cryptography, offer exponential speedups for solving complex Big Data problems, such as pattern recognition, optimization, and cryptography. Quantum Computing platforms, such as IBM Quantum and Google Quantum AI, enable researchers to experiment with quantum algorithms and explore their potential applications in Big Data analytics.
Conclusion:
In conclusion, Big Data technology represents a transformative force driving innovation, disruption, and value creation across industries and domains. leveraging advanced computing technologies, massive data sets, and sophisticated analytics tools, organizations can unlock the power of data to gain valuable insights, optimize operations, and drive strategic decision-making. addressing challenges related to data privacy, governance, and scalability, organizations can harness the full potential of Big Data technology to unlock new opportunities and shape the future of the digital age.