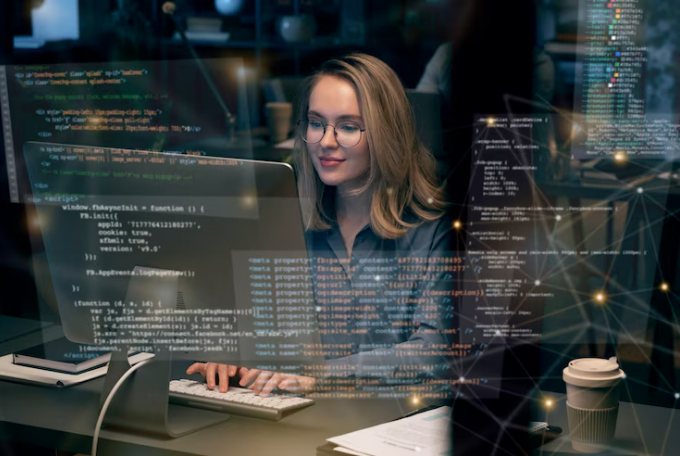
Introduction:
In the realm of technological advancement, Machine Learning (ML) stands as a beacon of innovation, continuously evolving to tackle increasingly complex challenges and redefine the boundaries of what’s possible. Within the vast landscape of ML techniques, certain approaches stand out as the most advanced, pushing the envelope of capabilities and enabling unprecedented feats of intelligence and automation. In this exploration of Machine Most Learning, we delve into these cutting-edge techniques, their applications, challenges, and the transformative impact they have on industries and society.
Advanced Techniques in Machine Most Learning:
- Deep Learning: At the forefront of Machine Most Learning is Deep Learning, a subset of ML that utilizes artificial neural networks with multiple layers (deep architectures) to extract high-level features from raw data. Deep Learning has revolutionized fields such as computer vision, natural language processing, and speech recognition, achieving human-level performance on tasks previously considered insurmountable. Convolutional Neural Networks (CNNs) excel at image recognition and object detection, while Recurrent Neural Networks (RNNs) are proficient in sequential data analysis. Transformer architectures, such as the BERT model, have pushed the boundaries of natural language understanding and generation.
- Generative Adversarial Networks (GANs): Generative Adversarial Networks (GANs) represent a breakthrough in the realm of generative modeling, enabling the creation of synthetic data that closely resembles real data distributions. GANs consist of two neural networks—the generator and the discriminator—competing in a game-theoretic framework. The generator generates synthetic data samples, while the discriminator tries to distinguish between real and synthetic samples. Through adversarial training, GANs learn to generate increasingly realistic and diverse data, with applications ranging from image synthesis and style transfer to drug discovery and anomaly detection.
- Reinforcement Learning: Reinforcement Learning (RL) has emerged as a powerful paradigm for training intelligent agents to make sequential decisions in dynamic environments. Unlike supervised learning, where models learn from labeled data, and unsupervised learning, where models learn patterns from unlabeled data, RL agents learn through trial and error, receiving feedback in the form of rewards or penalties based on their actions. Deep Reinforcement Learning combines deep neural networks with RL algorithms, enabling agents to learn complex behaviors and strategies. Applications of RL include robotics, autonomous vehicles, game playing, and recommendation systems.
- Transfer Learning: Transfer Learning addresses the challenge of training ML models with limited labeled data by leveraging knowledge gained from pre-trained models on large datasets. Instead of training a model from scratch, transfer learning fine-tunes a pre-trained model on a target task or domain, adapting its learned representations to new data. Transfer Learning has applications in domains where labeled data is scarce or expensive to obtain, such as medical imaging, natural language processing, and sensor data analysis. By transferring knowledge from related tasks, transfer learning accelerates model training, improves generalization, and reduces the need for large annotated datasets.
Applications of Machine Most Learning:
- Autonomous Systems: Machine Most Learning techniques are instrumental in developing autonomous systems that can perceive, reason, and act in complex and dynamic environments. Autonomous vehicles, such as self-driving cars and drones, rely on Deep Reinforcement Learning to navigate safely and efficiently, while robotic systems use GANs for sim-to-real transfer and domain adaptation. Machine Most Learning enables autonomous systems to learn from experience, adapt to changing conditions, and make decisions in real-time, paving the way for a future with intelligent and adaptive machines.
- Healthcare: In healthcare, Machine Most Learning techniques have the potential to revolutionize diagnosis, treatment, and patient care. Deep Learning models trained on medical imaging data, such as X-rays, MRIs, and CT scans, can assist radiologists in detecting abnormalities and predicting disease outcomes. GANs generate synthetic medical images for training data augmentation, while Reinforcement Learning optimizes treatment plans and drug dosages based on patient responses. Transfer Learning facilitates the adaptation of pre-trained models to specific medical specialties and conditions, accelerating model development and deployment in clinical settings.
- Natural Language Processing (NLP): Advancements in Machine Most Learning have propelled the field of Natural Language Processing (NLP) to new heights, enabling machines to understand, generate, and interact with human language in more sophisticated ways. Transformer-based models, such as GPT (Generative Pre-trained Transformer) and BERT (Bidirectional Encoder Representations from Transformers), have achieved state-of-the-art performance on a wide range of NLP tasks, including language translation, sentiment analysis, and question answering. GANs are used for text generation and style transfer, while Reinforcement Learning optimizes dialogue systems and conversational agents.
Challenges and Considerations in Machine Most Learning:
- Computational Complexity: The computational demands of Machine Most Learning techniques, especially Deep Learning and GANs, can be substantial, requiring large-scale parallel processing and specialized hardware accelerators. Training deep neural networks on massive datasets may involve weeks or even months of computation time, making scalability and resource efficiency critical challenges. Cloud computing platforms, distributed training frameworks, and hardware innovations such as GPUs and TPUs address these challenges to some extent but require ongoing optimization and investment.
- Data Quality and Bias: Machine Most Learning techniques are highly data-driven, relying on large volumes of high-quality data for training and evaluation. Data quality issues, such as noise, bias, and imbalance, can negatively impact model performance and generalization. Moreover, biased data can lead to biased predictions and discriminatory outcomes, particularly in sensitive domains such as healthcare and criminal justice. Addressing data quality and bias requires careful data collection, preprocessing, and model evaluation techniques to ensure fairness, transparency, and accountability.
- Interpretability and Explainability: The inherent complexity of Machine Most Learning models, particularly Deep Learning models and GANs, poses challenges for model interpretability and explainability. Understanding how these models make decisions is crucial for building trust, ensuring accountability, and identifying potential biases or errors. Techniques such as attention mechanisms, saliency maps, and adversarial attacks help interpret and explain model predictions, but achieving true transparency and interpretability remains an ongoing research challenge.
Future Directions in Machine Most Learning:
- Continual Learning: Continual Learning addresses the challenge of model forgetting and catastrophic interference in Machine Most Learning systems by enabling models to adapt and learn from new data over time. Unlike traditional batch learning, where models are trained offline on fixed datasets, continual learning algorithms incrementally update models with new data while preserving knowledge learned from previous experiences. Continual learning has applications in lifelong learning, adaptive systems, and autonomous agents operating in dynamic environments.
- Meta-Learning: Meta-Learning, or learning to learn, aims to develop ML models that can quickly adapt to new tasks or environments with minimal training data. Meta-learning algorithms learn from a distribution of tasks and examples to acquire meta-knowledge or meta-parameters that enable rapid adaptation to new tasks. Meta-learning has applications in few-shot learning, transfer learning, and domain adaptation, where models need to generalize across diverse datasets and environments with limited labeled data.
- Robust and Ethical AI: As Machine Most Learning techniques become more pervasive and autonomous, ensuring the robustness, fairness, and ethical behavior of AI systems becomes paramount. Robust AI techniques address vulnerabilities such as adversarial attacks, data poisoning, and distributional shifts that can compromise the reliability and safety of ML models. Ethical AI frameworks promote transparency, accountability, and inclusivity in AI development and deployment, safeguarding against biases, discrimination, and unintended consequences.
Conclusion:
In conclusion, Machine Most Learning represents the pinnacle of technological innovation, pushing the boundaries of intelligence, automation, and creativity. Through advanced techniques such as Deep Learning, GANs, Reinforcement Learning, and Transfer Learning, Machine Most Learning enables machines to learn, reason, and adapt in ways that were once thought impossible. From autonomous systems and healthcare to natural language processing and beyond, Machine Most Learning is transforming industries, driving innovation, and shaping the future of humanity. As we continue to explore the frontiers of Machine Most Learning, let us embrace its potential to solve complex challenges, enhance human capabilities, and build a better, more intelligent world for generations to come.