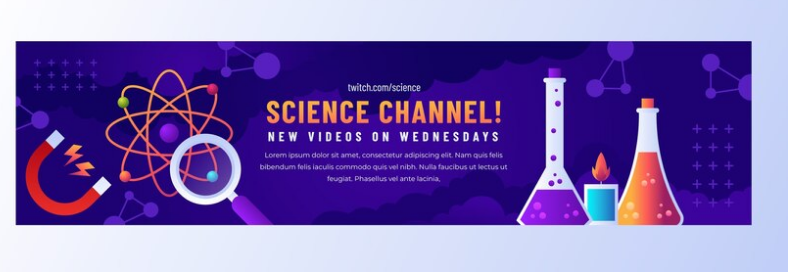
Introduction:
In the dynamic landscape of technology, the fusion of Big Data and Network Technology has emerged as a catalyst for innovation, enabling organizations to leverage vast amounts of data flowing through interconnected networks to drive insights, optimize operations, and fuel strategic decision-making. The convergence of Big Data analytics and network technologies empowers organizations to extract valuable insights from network traffic, device interactions, and user behavior, enabling them to enhance cybersecurity, improve network performance, and deliver personalized experiences. In this exploration of Big Technology Network Data, we delve into the underlying principles, applications, challenges, and future prospects of this transformative synergy.
Understanding Big Technology Network Data:
- Big Data in Networking: Big Data in networking refers to the collection, storage, processing, and analysis of large volumes of network-related data, including network traffic, device logs, and telemetry data. Network devices such as routers, switches, and firewalls generate vast amounts of data about packet flows, bandwidth utilization, and network performance metrics. By capturing and analyzing this data, organizations gain insights into network behavior, identify anomalies, and troubleshoot performance issues in real-time. Big Data analytics platforms ingest and process network data streams to detect patterns, trends, and security threats, enabling proactive network management and optimization.
- Network Technologies for Big Data: Network technologies play a critical role in facilitating the exchange of data between distributed systems and enabling seamless communication and collaboration in Big Data environments. High-speed networks, such as Ethernet and fiber optic networks, provide the bandwidth and low latency required for transferring large volumes of data between data centers, cloud platforms, and edge devices. Network protocols, such as TCP/IP, HTTP, and MQTT, govern data transmission and ensure reliable delivery of data packets across heterogeneous networks. Software-defined networking (SDN) and network virtualization technologies abstract network resources and enable programmable network management, facilitating dynamic allocation of bandwidth and prioritization of traffic based on application requirements.
- Convergence of Big Data and Networking: The convergence of Big Data and networking technologies enables organizations to extract actionable insights from network data and leverage them to enhance cybersecurity, optimize network performance, and deliver personalized services. Big Data analytics platforms analyze network traffic patterns, detect anomalies, and correlate events across disparate data sources to identify security threats, such as malware infections, DDoS attacks, and insider threats. Network monitoring and management tools leverage real-time analytics to optimize bandwidth utilization, predict network congestion, and prioritize critical applications based on traffic patterns and user behavior.
Applications of Big Technology Network Data:
- Cybersecurity and Threat Intelligence: In the realm of cybersecurity, Big Technology Network Data empowers organizations to detect and mitigate security threats proactively. Network traffic analysis platforms monitor network traffic in real-time, identify suspicious activities, and generate alerts for potential security incidents. Machine learning algorithms analyze network data patterns and user behavior to detect advanced threats, such as zero-day exploits and insider threats, and classify security events based on risk severity. Threat intelligence feeds provide organizations with actionable insights into emerging cyber threats, enabling them to strengthen their defenses and protect sensitive data assets.
- Network Performance Optimization: Big Technology Network Data enables organizations to optimize network performance and ensure seamless connectivity for mission-critical applications and services. Network monitoring tools capture and analyze network traffic patterns, identify bottlenecks, and measure network latency and packet loss rates. Predictive analytics algorithms forecast network traffic demand, predict bandwidth requirements, and optimize network routing to reduce congestion and improve application performance. Quality of Service (QoS) policies prioritize critical applications and allocate bandwidth dynamically based on application requirements, ensuring optimal user experience and service delivery.
- Personalized Customer Experiences: In the realm of customer engagement, Big Technology Network Data enables organizations to deliver personalized experiences and tailor products and services to individual preferences. Customer relationship management (CRM) systems capture and analyze customer interactions across multiple channels, including websites, mobile apps, and social media platforms. Big Data analytics platforms analyze customer data, including browsing behavior, purchase history, and demographic information, to segment customers into targeted audience segments and personalize marketing campaigns and product recommendations. Real-time analytics enable organizations to deliver context-aware experiences and engage customers at the right time and through the right channels.
Challenges and Considerations in Big Technology Network Data:
- Data Privacy and Compliance: As organizations capture and analyze network data containing sensitive information, protecting data privacy and ensuring regulatory compliance become paramount concerns. Data protection regulations, such as GDPR and CCPA, impose strict requirements on organizations to safeguard personal data and respect user privacy rights. Compliance with data privacy regulations requires organizations to implement robust data encryption, access controls, and data anonymization techniques to protect sensitive information from unauthorized access or disclosure.
- Scalability and Performance: Scalability and performance are critical considerations in Big Technology Network Data, as organizations strive to process and analyze large volumes of network data in real-time. Traditional network monitoring tools may struggle to scale horizontally and handle the high throughput and low latency requirements of Big Data analytics. Distributed stream processing frameworks, such as Apache Kafka and Apache Flink, enable organizations to ingest and process data streams from multiple sources in parallel, ensuring timely detection and response to security threats and performance issues.
- Integration and Interoperability: Integrating Big Data analytics platforms with existing network infrastructure and security tools requires careful planning and coordination to ensure seamless data exchange and interoperability. Compatibility issues between data formats, protocols, and APIs may hinder the integration process and impede the flow of data between disparate systems. Open standards and APIs facilitate interoperability between Big Data analytics platforms, network devices, and security appliances, enabling organizations to leverage the full potential of Big Technology Network Data and extract maximum value from their investments.
Future Prospects in Big Technology Network Data:
- Artificial Intelligence and Automation: The integration of Artificial Intelligence (AI) and automation technologies with Big Technology Network Data enables organizations to enhance threat detection, automate incident response, and optimize network operations. AI-driven network security solutions leverage machine learning algorithms to analyze network data patterns, detect anomalies, and adapt to evolving threats in real-time. Autonomous network management platforms use AI-driven analytics to predict network failures, automate network configuration, and optimize resource allocation based on changing traffic patterns and user demands.
- Edge Computing and Distributed Analytics: The proliferation of edge computing and IoT devices generates vast amounts of data at the network edge, posing challenges for data processing and analysis in centralized data centers. Edge computing platforms bring compute resources closer to data sources, enabling real-time analytics and decision-making at the network edge. Distributed analytics frameworks, such as federated learning and edge-native analytics, enable organizations to distribute data processing tasks across edge devices and aggregate insights from distributed data sources without compromising data privacy or security.
- Quantum Networking and Cryptography: The emergence of quantum networking and cryptography holds promise for revolutionizing network security and data encryption in the era of Big Technology Network Data. Quantum key distribution (QKD) protocols enable secure communication channels between network nodes by leveraging the principles of quantum mechanics to generate unbreakable encryption keys. Quantum networking technologies, such as quantum repeaters and quantum routers, enable quantum entanglement and teleportation of data packets over long distances, paving the way for ultra-secure and high-speed communication networks resistant to quantum attacks.
Conclusion:
In conclusion, the fusion of Big Data and Network Technology represents a paradigm shift in how organizations capture, analyze, and leverage data to drive insights, optimize operations, and deliver personalized experiences. By harnessing the power of Big Technology Network Data, organizations can enhance cybersecurity, improve network performance, and unlock new opportunities for innovation and growth. By addressing challenges related to data privacy, scalability, and interoperability, organizations can realize the full potential of Big Technology Network Data and shape the future of digital transformation in the interconnected world.